About Me
I am currently a Postdoctoral Research Fellow in the Department of Electrical and Computer Engineering at University of Alberta, under the mentorship of Prof. Lei Ma and Prof. Zhan Shu. I received my Ph.D. from the Technical University of Munich in 2022, where I specialized in automatic control and robotics under the supervision of Prof. Martin Buss.
My research interests include learning-based control, safe reinforcement learning, Artificial Intelligence (AI) and Machine Learning (ML) approaches, and their applications in robotics and cyber-physical systems. I am focused on developing intelligent and robust AI-enabled autonomous and robotic systems with safety and reliability guarantees, ensuring their applicability to a wide range of complex real-world tasks and scenarios.
News
- 01/2025: Our paper “Multilingual Blending: LLM Safety Alignment Evaluation with Language Mixture” was accpeted by NAACL 2025!
- 11/2024: Our paper “GenSafe: A Generalizable Safety Enhancer for Safe Reinforcement Learning Algorithms Based on Reduced Order Markov Decision Process Model” was published on IEEE Transactions on Neural Networks and Learning Systems!
- 06/2024: We gave a tutorial on “Quality Assurance for Autonomous Driving Systems: A Software Engineering Perspective” at Autoware Tutorial, IEEE IV 2024.
- 05/2024: Our paper “ISR-LLM: Iterative Self-Refined Large Language Model for Long-Horizon Sequential Task Planning” was presented at IEEE ICRA 2024!
- 03/2024: Our paper “Towards Building AI-CPS with Nvidia Isaac Sim: An Industrial Benchmark and Case Study for Robotics Manipulation” was presented at IEEE/ACM ICSE-SEIP 2024!
- 02/2024: Our paper “Enabling Versatility and Dexterity of the Dual-Arm Manipulators: A General Framework Toward Universal Cooperative Manipulation” was published on IEEE Transactions on Robotics!
- 10/2022: Our paper “Off-Policy Risk-Sensitive Reinforcement Learning-Based Constrained Robust Optimal Control” was published on IEEE Transactions on Systems, Man, and Cybernetics: Systems!
- 09/2022: I gave a talk on “Safe Reinforcement Learning with Model Order Reduction Techniques” at The 1st International Workshop on Safe Reinforcement Learning Theory and its Applications, IEEE MFI 2022.
- 07/2022: I joined the Department of Electrical and Computer Engineering at University of Alberta as a Postdoctoral Research Fellow.
- 07/2022: I received my Ph.D. degree from the Chair of Automatic Control Engineering (LSR) at the Technical University of Munich (TUM), supervised by Prof. Martin Buss.
- 09/2021: Our paper “Learning a Low-Dimensional Representation of a Safe Region for Safe Reinforcement Learning on Dynamical Systems” was published on IEEE Transactions on Neural Networks and Learning Systems!
- 06/2020: Our paper “A General Framework to Increase Safety of Learning Algorithms for Dynamical Systems Based on Region of Attraction Estimation” was published on IEEE Transactions on Robotics!
Featured Publications
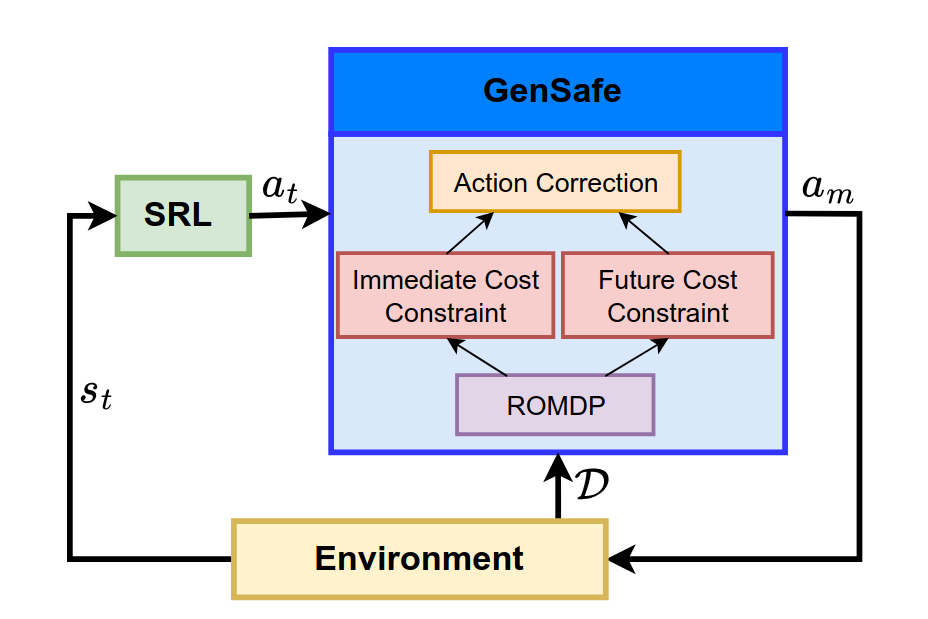
GenSafe: A Generalizable Safety Enhancer for Safe Reinforcement Learning Algorithms Based on Reduced Order Markov Decision Process Model
Zhehua Zhou, Xuan Xie, Jiayang Song, Zhan Shu, Lei Ma
IEEE Transactions on Neural Networks and Learning Systems, November, 2024
In this work, we introduce a Genralizable Safety enhancer (GenSafe) to improve the safety performance of safe reinforcement learning algorithms.
PDF
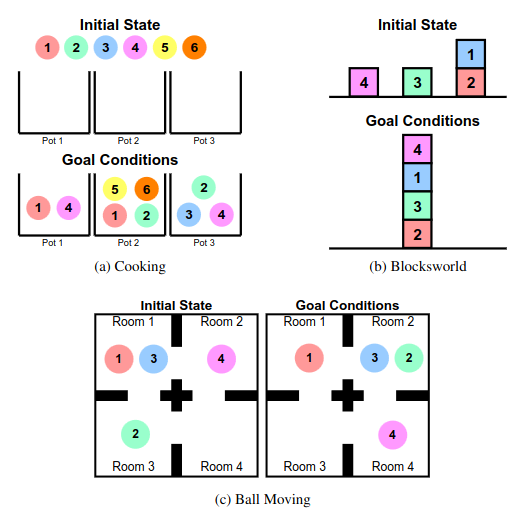
ISR-LLM: Iterative Self-Refined Large Language Model for Long-Horizon Sequential Task Planning
Zhehua Zhou, Jiayang Song, Kunpeng Yao, Zhan Shu, Lei Ma
2024 IEEE International Conference on Robotics and Automation (ICRA), May, 2024
In this work, we introduce ISR-LLM, a novel framework that improves LLM-based planning through an iterative self-refinement process.
PDF
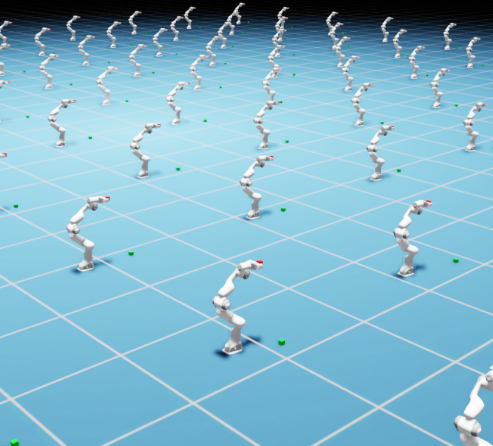
Towards Building AI-CPS with NVIDIA Isaac Sim: An Industrial Benchmark and Case Study for Robotics Manipulation
Zhehua Zhou, Jiayang Song, Xuan Xie, Zhan Shu, Lei Ma, Dikai Liu, Jianxiong Yin, Simon See
IEEE/ACM 46th International Conference on Software Engineering: Software Engineering in Practice (ICSE-SEIP), April, 2024
As a foundational step towards building reliable AI-enabled robotics systems, in this paper, we propose a public benchmark for robotics manipulation.
PDF
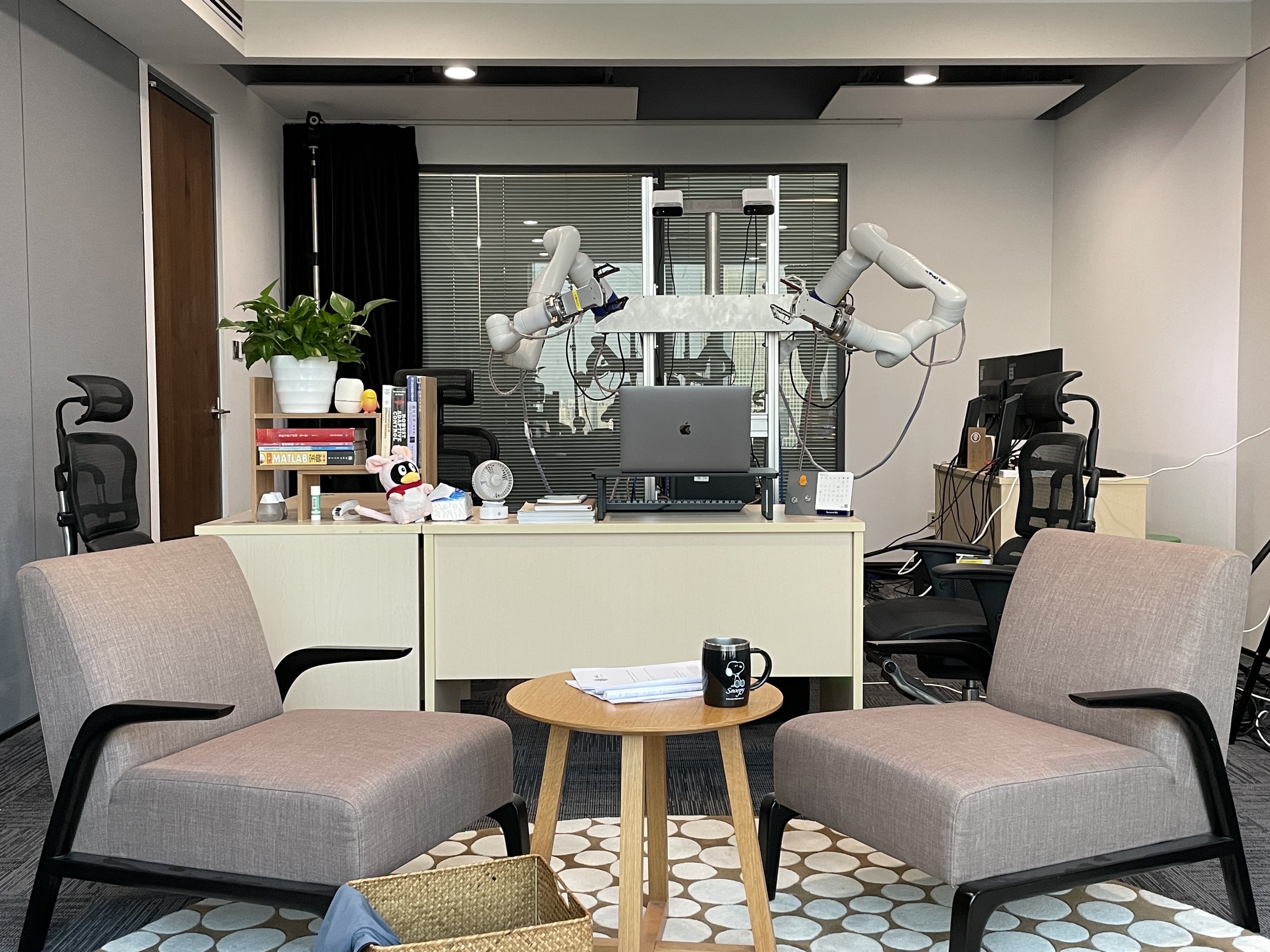
Enabling Versatility and Dexterity of the Dual-Arm Manipulators: A General Framework Toward Universal Cooperative Manipulation
Yi Ren*, Zhehua Zhou* (equal contribution), Ziwei Xu, Yang Yang, Guangyao Zhai, Marion Leibold, Fenglei Ni, Zhengyou Zhang, Martin Buss, Yu Zheng
IEEE Transactions on Robotics, February, 2024
In this article, we propose a general and versatile control framework for dual-arm manipulators.
PDF
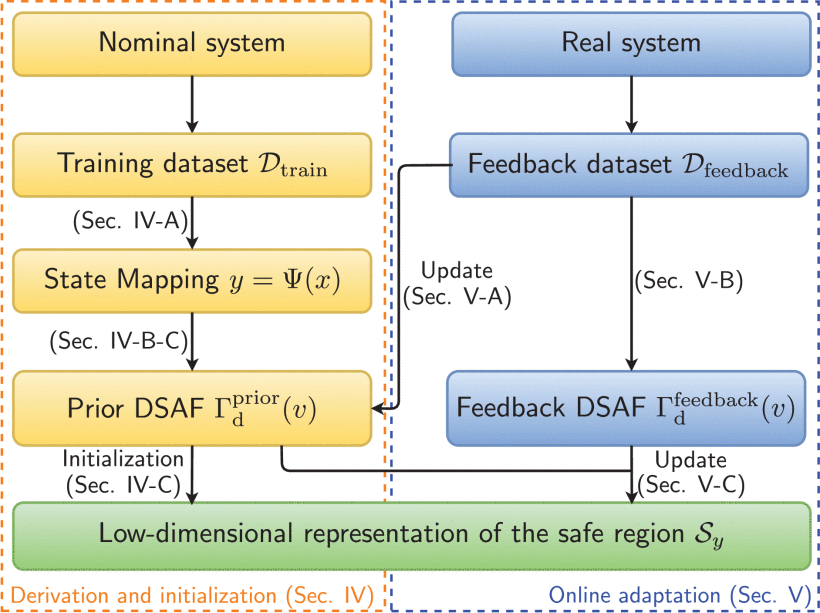
Learning a Low-Dimensional Representation of a Safe Region for Safe Reinforcement Learning on Dynamical Systems
Zhehua Zhou, Ozgur S Oguz, Marion Leibold, Martin Buss
IEEE Transactions on Neural Networks and Learning Systems, September, 2021
In this article, we propose a general data-driven model order reduction approach for safe reinforcement learning.
PDF
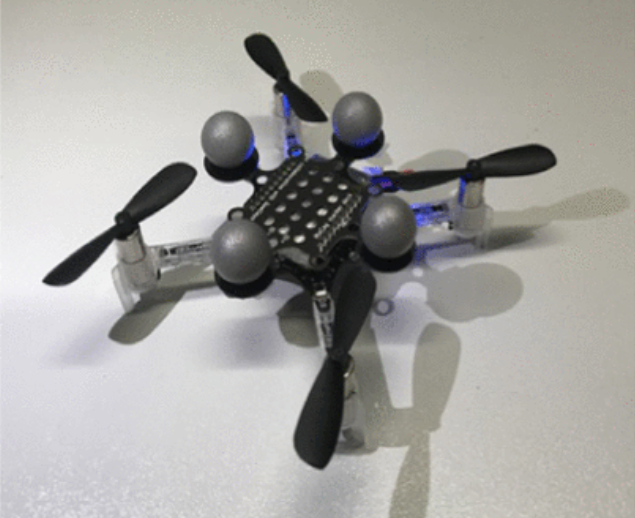
A General Framework to Increase Safety of Learning Algorithms for Dynamical Systems Based on Region of Attraction Estimation
Zhehua Zhou, Ozgur S Oguz, Marion Leibold, Martin Buss
IEEE Transactions on Robotics, June, 2020
In this article, we propose a computationally effective and general safe learning framework, specifically for complex dynamical systems.
PDF
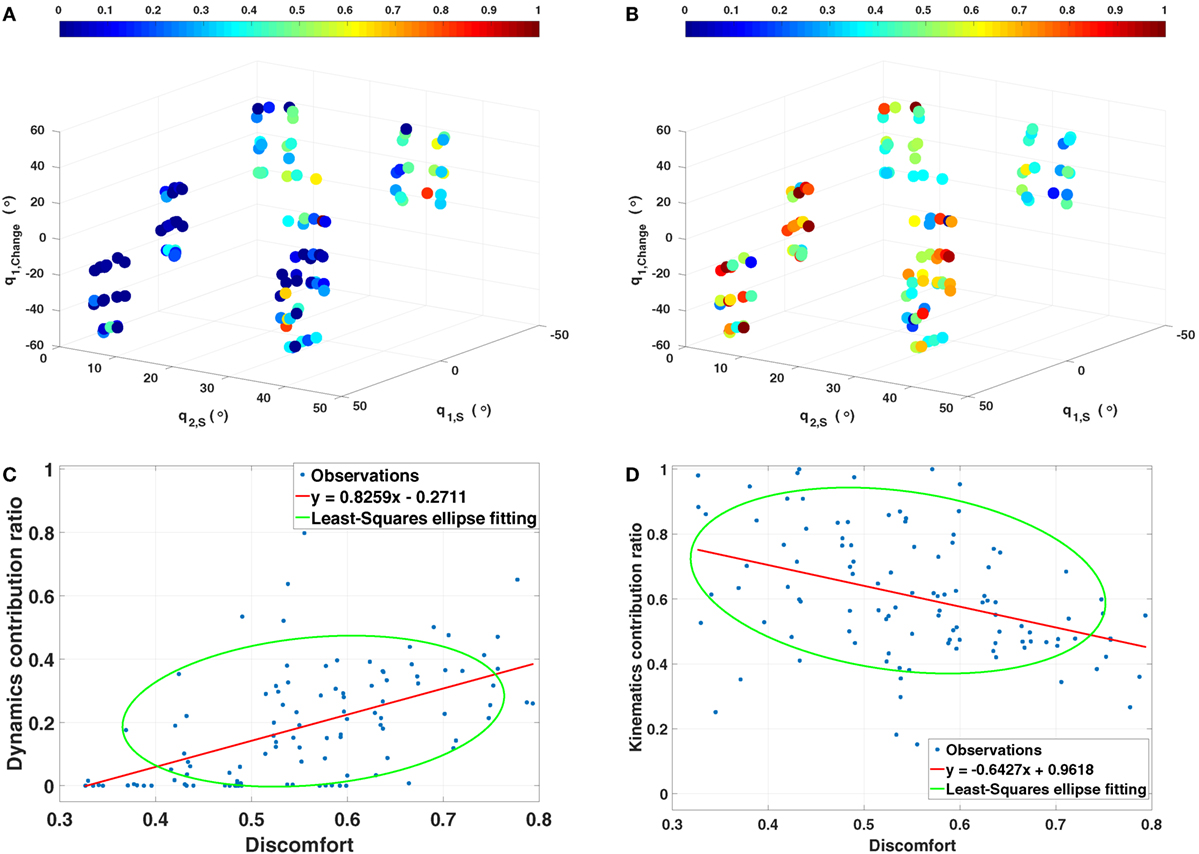
A Hybrid Framework for Understanding and Predicting Human Reaching Motions
Ozgur S Oguz, Zhehua Zhou, Dirk Wollherr
Frontiers in Robotics and AI, March, 2018
In this work, we propose a hybrid framework for understanding and predicting human reaching motions for human-robot collaboration.
PDF